Challenges: Climate predictions aim to estimate the future evolution of climate on sub-seasonal to decadal time scales. Forecast systems (i.e., initialized climate models) are essential tools in climate prediction and their correct initialization, which synchronizes the model climate with the real climate, is necessary to exploit the real predictability of the climate system. Knowledge of the climate state at a given point in time is only possible through extensive observations of the different components of the climate system. Furthermore, to correctly evaluate past forecasts and improve the systems, continuous observations in time and space are necessary. This “big picture” view of most of the planet has only been available since satellites became operational in the late 1970s. Additionally, deployment of complementary in-situ observing systems has significantly increased in the past decades, filling important gaps, and as a result improving forecast quality.
The different components of the climate systems interact and have characteristic timescales of predictability. The ocean is the most important component on long timescales (over months to decades), while the atmosphere is critical on short timescales (hours to a week). The land and the sea ice state contain information useful mostly on intermediate timescales (weeks to over a seasons). Hence initializing the key variables of the sea ice component may be beneficial for seasonal and decadal predictions. However, sea-ice initialization is not yet a standard practice in seasonal-to-decadal climate prediction. Sea ice concentration (SIC) and sea ice thickness (SIT) are thought to be fundamental variables to initialize sea ice in fully coupled models.
Another example of particular relevance for various users is to predict (up to 10 days ahead) and monitor fresh water inflow to the Arctic Ocean and changes in Arctic hydrological regimes for the major Arctic rivers. This is done by employing the hydrological model Arctic-HYPE (see http://hypeweb.smhi.se). The challenge behind this effort can be illustrated by the pure description of the spatial dimensions with a model domain covering 26 Mkm² distributed over 34421 sub-basins (see map below).
Results obtained in INTAROS: The above-mentioned Arctic-HYCOS river discharges compiled at SMHI are part of the iAOS and have been assimilated to produce a pan-Arctic hydrological analyses and subsequent forecasts with the Arctic-HYPE model. The functionality of this workflow has been demonstrated via a use-case in the Republic of Sacha (Yakutia), in Far-East Russia, where a sub-set of the Arctic-HYPE model was successfully used for spring flood and river ice breakup forecasting in the major Yakutia rivers.
Evaluations of sensitivity studies regarding retrospective EC-Earth3-based climate forecasts (hindcasts) performed by SMHI in collaboration with the Danish Meteorological Institute for the period 1996-2016 show that the initialization of sea-ice concentrations and in particular sea-ice thickness is in general beneficial for seasonal and decadal prediction skill over the Arctic region (see fig below and Tian et al., 2021). The CERSAT dataset on Arctic sea-ice concentrations - produced within INTAROS and part of the iAOS - has been used as independent reference for validation of these climate predictions as well as for assimilation experiments meant to pave the way for the next generation of decadal climate predictions at SMHI.
The same CERSAT sea-ice concentrations have been assimilated for BSC’s seasonal climate prediction system (also employing EC-Earth3). It is shown that the assimilation of sea-ice concentrations does not yield significant benefit for winter seasonal predictions (started on 1 November) but do have a remarkable positive impact on summer seasonal predictions (started on 1 May) regarding the sea-ice edge but also remote North Atlantic SSTs. The latter is shown to be the result of a so-called atmospheric bridge translating the improved sea-ice representation via more realistic large-scale atmospheric variability into the SST-signal.
Anomalies derived from sea-ice concentrations as well as C2SMOS and ENVISAT CCI sea-ice thickness estimates were assimilated in NorCPM, the seasonal-to-decadal climate prediction system developed at NERSC. It is shown that the assimilation of sea-ice concentrations is particularly beneficial for predictions along the sea-ice edge while sea-ice thickness is more important for the central Arctic. Hence, the assimilation of both is complementary and yields the best overall result. Here, the assimilation of C2SMOS data provides significantly better results compared to ENVISAT CCI.
Three partners (SMHI, BSC and NERSC) contribute to the CMIP6 Decadal Climate Prediction Project (DCPP). Respective climate prediction data is freely available via the Earth System Grid Federation. BSC and SMHI further participate in the WMO exchange of annual-to-decadal climate predictions (https://hadleyserver.metoffice.gov.uk/wmolc/). Thus, all partners actively distribute knowledge and results produced in INTAROS within the wider community of climate prediction modeling. The prediction data itself, including SMHI’s hydrological forecasts is used for various climate services, climate impact studies, as well as economic models.
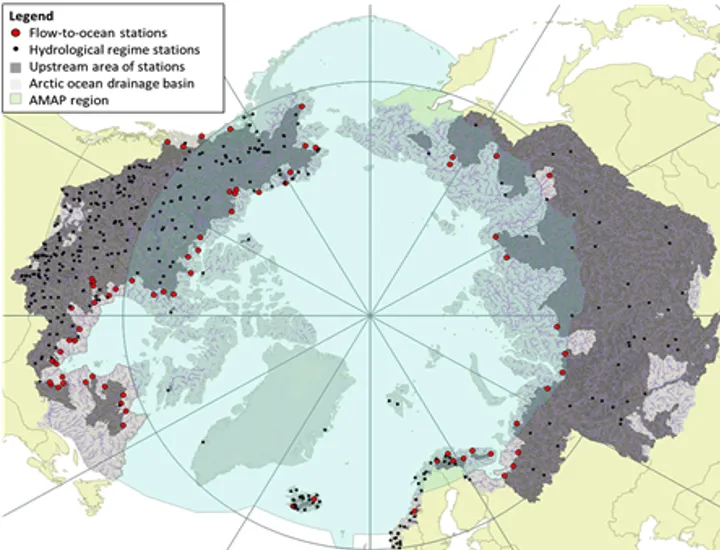
Map of drainage basin of the Arctic Ocean and related water bodies in the northern seas as represented in the Arctic-HYPE model (light grey), the location of the Arctic-HYCOS stations and their upstream drainage basins (dark grey).
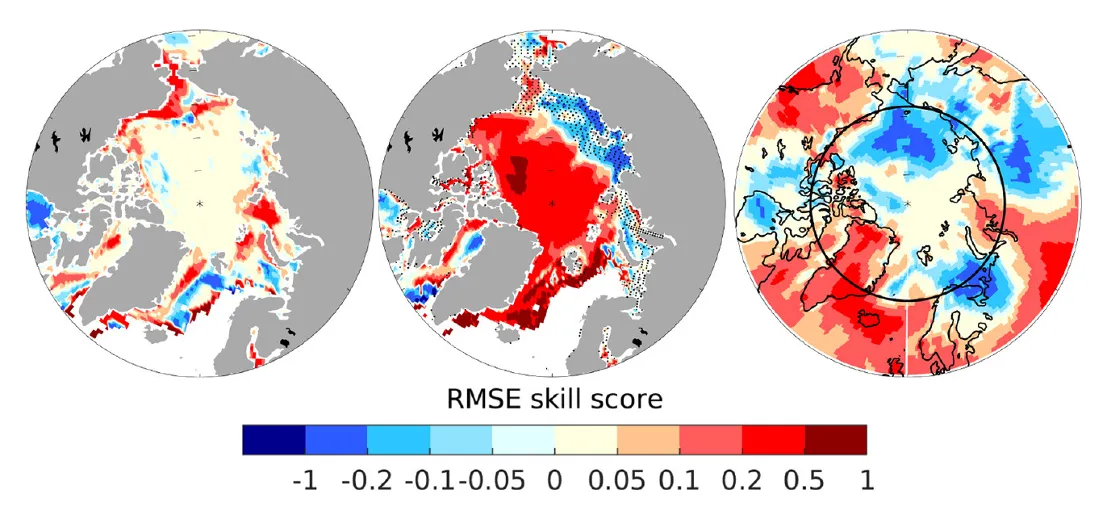
Seasonal prediction skill (measured by the RMSE skill score) gained by the initialization of sea-ice variables for the first winter (DJF) average of sea-ice concentrations (left), sea-ice thickness (center), and 2m-temperature (right). Positive values indicate higher skill (smaller forecast error) than when initializing only ocean temperature and salinity. Figure taken from Tian et al. (2021; https://doi.org/10.5194/gmd-14-4283-2021, distributed under the Creative Commons Attribution 4.0 License).
Contributing partners:
Swedish Meteorological and Hydrological Institute (SMHI), Barcelona Supercomputing Centre (BSC), Nansen Environmental and Remote Sensing Centre (NERSC), University of Hamburg (UHAM).
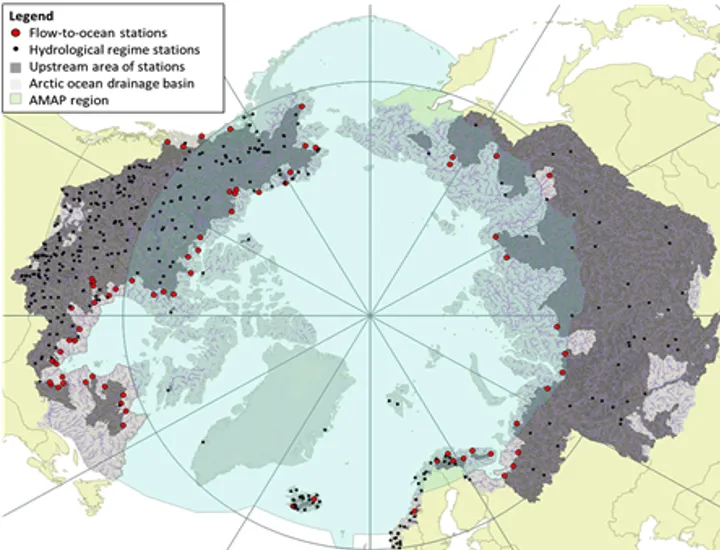
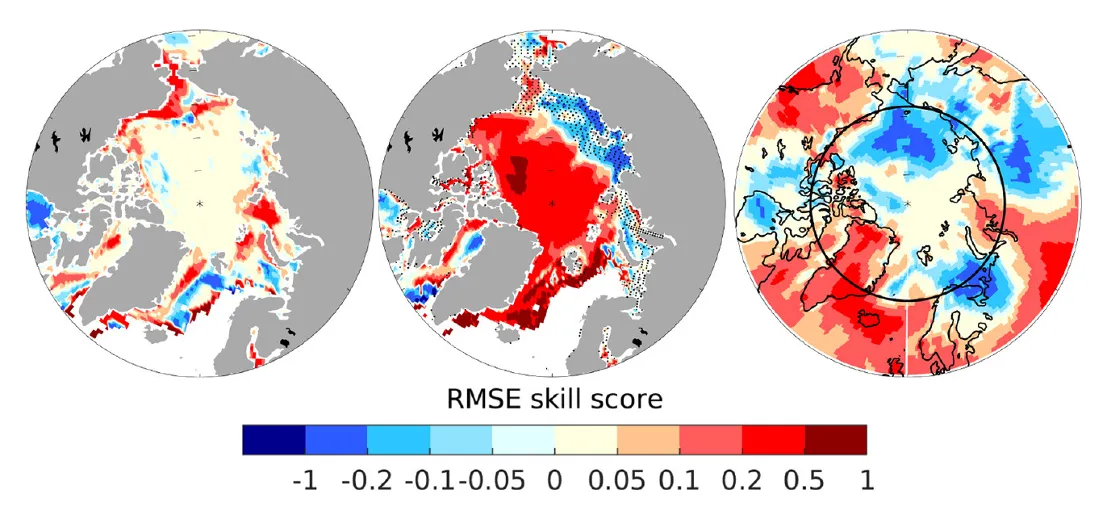